October 31, 2023 at 8:35 pm | Updated November 6, 2023 at 4:46 pm | 5 min read
Welcome to another exciting blog post where we explore the cutting-edge research that’s shaping the future of agriculture. Today, we’re diving into a fascinating study titled “Multiscale Inversion of Leaf Area Index in Citrus Tree by Merging UAV LiDAR with Multispectral Remote Sensing Data.” Conducted by a team of researchers led by Weicheng Xu and Feifan Yang, this study holds significant promise for the field of agricultural remote sensing. In this blog post, we’ll not only unpack the research but also highlight the role played by one of CID Bio-Science’s very own devices in making this study a success.
Research Objectives
The primary objective of this research was to develop a machine-learning model capable of estimating the Leaf Area Index (LAI) in citrus orchards. The researchers used a combination of UAV LiDAR and multispectral data to achieve this. Why is this important, you ask? Accurate LAI estimation is crucial for agricultural monitoring and decision-making, affecting everything from irrigation to pest management.
The Evolving Landscape of UAVs in Agriculture
The Current State of UAV Remote Sensing in Agriculture
Subscribe to the CID Bio-Science Weekly article series.
By submitting this form, you are consenting to receive marketing emails from: . You can revoke your consent to receive emails at any time by using the SafeUnsubscribe® link, found at the bottom of every email. Emails are serviced by Constant Contact
Unmanned Aerial Vehicles (UAVs) have been a hot topic in agricultural research for a while now. They offer a range of benefits, from high-resolution imaging to real-time data collection. However, the field continuously evolves, and researchers constantly look for ways to improve accuracy and efficiency.
To give you a broader perspective, let’s consider another study titled “Deep Learning Techniques to Classify Agricultural Crops through UAV Imagery: a review.” This research aimed to review the effectiveness of deep learning techniques in classifying agricultural crops through UAV imagery. While the study found promising results, it also highlighted several challenges, such as the need for extensive and diverse datasets and the lack of standardization in data collection.
Why This Research is Important
The study we’re focusing on today takes UAV remote sensing a step further by merging it with multispectral data. This approach not only improves the accuracy of LAI estimation but also opens up new avenues for agricultural monitoring. It’s not just about collecting data; it’s about collecting the right kind of data and using it effectively. And that’s where our instrument comes into play, but more on that later.
Stay tuned as we delve into the research methodology and discuss how CID Bio-Science’s instrument contributed to this groundbreaking study.
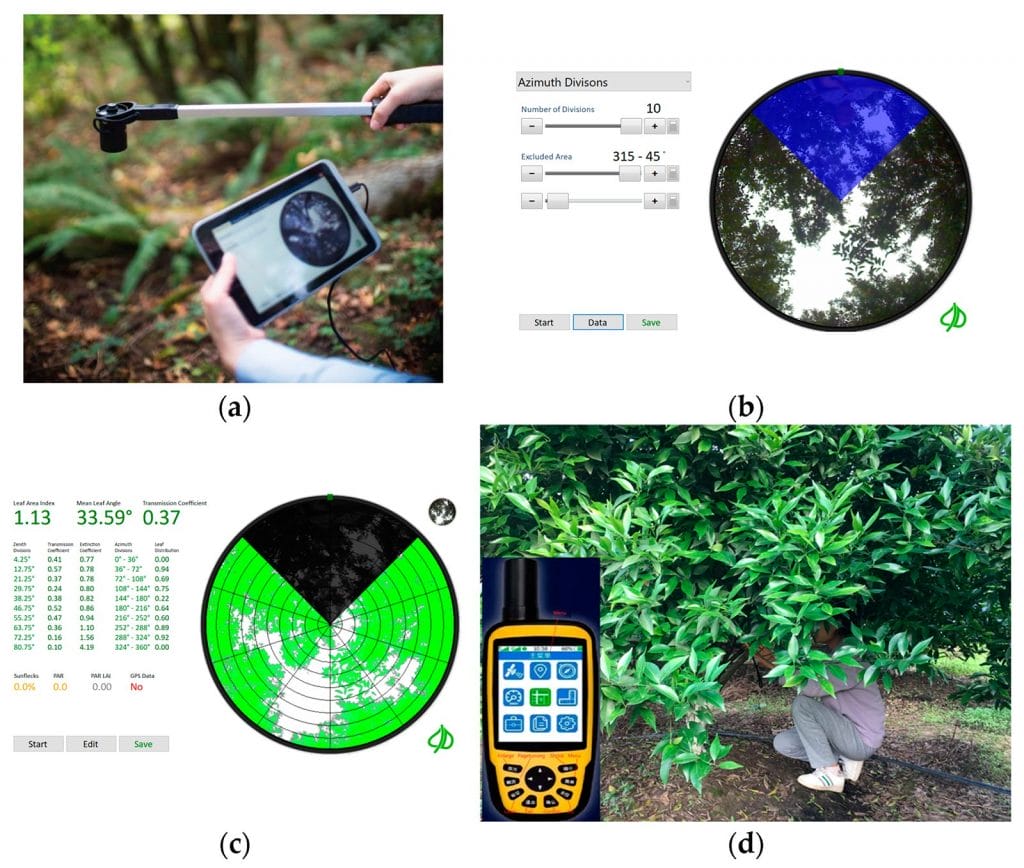
Research Methodology
In this study, the researchers employed a robust methodology to achieve their objectives. They utilized Unmanned Aerial Vehicles (UAVs) equipped with LiDAR and multispectral cameras. The data collected underwent preprocessing to remove noise and was then used to train a machine-learning model for LAI estimation.
Role of CID Bio-Science’s Instrument
Our instrument played a pivotal role in this research. It contributed to the data collection process by providing high-precision ground truth LAI. These measurements were integral in training the machine learning model, enhancing its accuracy and reliability in LAI estimation.
The Real-World Impact of Accurate LAI Estimation
Understanding the Leaf Area Index (LAI) is not just an academic exercise; it has real-world applications that can significantly impact agriculture and environmental science. Accurate LAI estimation aids in effective irrigation management, pest control, and yield prediction.
To provide a broader understanding, another study titled “An Overview of Global Leaf Area Index (LAI): Methods, Products, Validation, and Applications” highlights the importance of LAI in understanding the exchange of energy, water, and carbon between the land surface and the atmosphere. This paper also emphasizes the evolving nature of LAI estimation methods, including using various remote sensing data sources like optical, radar, and LiDAR data. The coefficient of determination (R^2) for LAI estimation using different methods was found to be greater than 0.72, affirming the reliability of these techniques.
Findings
The research findings of the study are noteworthy. The machine learning model developed effectively estimated LAI at both community and individual scales. The use of UAV LiDAR and multispectral data not only improved the accuracy but also provided a more comprehensive understanding of citrus orchards.
By merging these two data types, the researchers achieved a more accurate and reliable LAI estimation, contributing to the broader goals of agricultural monitoring and decision-making.
How The LAI Meter Elevates the Research
The LAI Meter from CID Bio-Science was not just a tool in this research; it was a cornerstone. Specializing in high-precision Leaf Area Index (LAI) measurements, our LAI Meter was integral to the data collection. Its unique features, such as rapid data acquisition and exceptional accuracy, made it indispensable for training the machine learning model used in the study.
By providing reliable and precise LAI measurements, our instrument significantly enhanced the model’s performance. This, in turn, contributed to the study’s overall success in achieving more accurate and reliable LAI estimations for citrus orchards.
Limitations and Future Research
While the study “Multiscale Inversion of Leaf Area Index in Citrus Tree by Merging UAV LiDAR with Multispectral Remote Sensing Data” achieved its primary objectives, it’s crucial to recognize its limitations for a well-rounded understanding. One notable constraint was the study’s focus on citrus orchards, which may limit the generalizability of the findings to other types of agricultural landscapes. Additionally, the research relied on a specific set of remote sensing technologies and a particular machine learning model, which could influence the applicability of the results in different contexts.
These limitations, however, offer fertile ground for future research. For instance, subsequent studies could aim to validate the machine learning model in diverse agricultural settings, such as vineyards or grain fields. There’s also potential for integrating other types of remote sensing data or employing different machine learning algorithms to improve LAI estimation accuracy further.
Essential Insights to Carry Forward
As we near the end of our exploration of this impactful research, let’s crystallize the essential points that readers should carry forward:
- Innovative Methodology: The research employed a novel approach by merging UAV LiDAR with multispectral data, setting a new standard in agricultural remote sensing.
- Significance of Accurate LAI Estimation: The study underscored the importance of accurate LAI estimation in agricultural monitoring and decision-making, particularly in areas like irrigation and pest control.
- Instrumental Role of CID Bio-Science’s LAI Meter: Our LAI Meter was not merely a data collection tool; it was a critical component that significantly enhanced the machine learning model’s performance in LAI estimation.
- Potential for Future Research: While the study had its limitations, it opened avenues for future research, including the exploration of different agricultural landscapes and the integration of various remote sensing technologies.
By understanding these key takeaways, readers can appreciate both the depth and breadth of the research, along with the significant role played by our LAI Meter in advancing the field.
Sources
Xu, W.; Yang, F.; Ma, G.; Wu, J.; Wu, J.; Lan, Y. Multiscale Inversion of Leaf Area Index in Citrus Tree by Merging UAV LiDAR with Multispectral Remote Sensing Data. Agronomy 2023, 13, 2747. https://doi.org/10.3390/agronomy13112747
Bouguettaya, A., Zarzour, H., Kechida, A. et al. Deep learning techniques to classify agricultural crops through UAV imagery: a review. Neural Comput & Applic 34, 9511–9536 (2022). https://doi.org/10.1007/s00521-022-07104-9
2019). An overview of global leaf area index (LAI): Methods, products, validation, and applications. Reviews of Geophysics. 57, 739–799. https://doi.org/10.1029/2018RG000608
, , , & (
Related Products
Most Popular Articles
- Transpiration in Plants: Its Importance and Applications
- Leaf Area – How & Why Measuring Leaf Area…
- How to Analyze Photosynthesis in Plants: Methods and Tools
- The Forest Canopy: Structure, Roles & Measurement
- Forest & Plant Canopy Analysis – Tools…
- Plant Respiration: Its Importance and Applications
- Stomatal Conductance: Functions, Measurement, and…
- Root Respiration: Importance and Applications
- The Importance of Leaf Area Index (LAI) in…
- Irrigating with Saline or Seawater